AI translation vs. machine translation
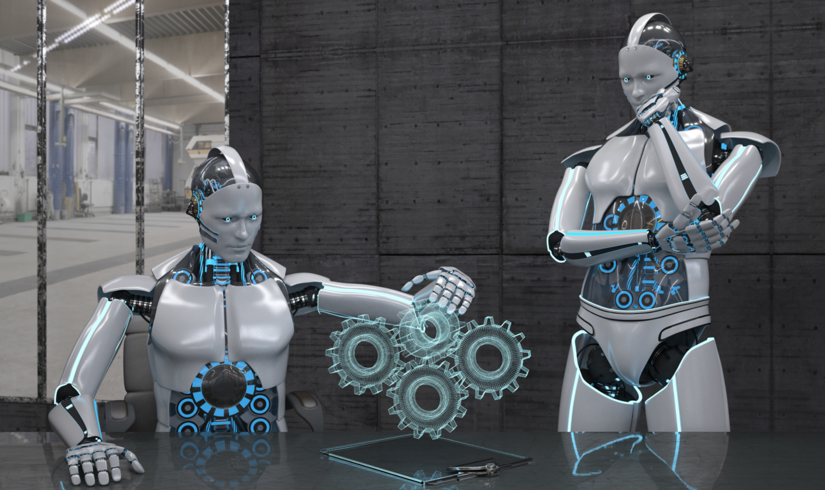
Artificial intelligence and the possibilities it enables are currently on everyone's lips, and its capabilities are also currently being tested in the translation industry. The term “AI translation” has quickly been coined, but what exactly is this and how does it differ from classic machine translation? And will it soon come to pass that medical texts are only translated using machines or artificial intelligence? In what follows, we will take a closer look.
What are AIs?
Artificial intelligence, or AI for short, can now be deployed in a wide variety of areas – text generation, graphics, programming, and also translation. AIs attempt to artificially reproduce human thinking and learning and transfer this to computers and machines. This should allow them to imitate human cognitive abilities. The goal is to develop machines that learn and thus become more intelligent and also more human. Artificial intelligence will give rise to machines that can independently complete tasks and solve problems. AIs can specialize in various subject areas, e.g., text or image generation.
The ChatGPT language model can, for example, write, optimize, and translate texts, research information, and provide support with organizational tasks. When you ask ChatGPT about the types of tasks that it can complete, it will provide you with a clear list, including its limitations.

DALL.E, on the other hand, can create images from language prompts. The more specific the prompt, the more precise the result. As an example, we asked DALL.E to create an image of a translator at work on a project.

What are the industries where AIs can be deployed?
The areas of application of AIs are wide-ranging. In everyday working life, there are now a wide variety of fields where artificial support can be deployed, including:
- Marketing: Text and graphics, brainstorming
- Business: Business intelligence
- IT: Programming
- Mathematics: Solving math problems
- Automotive: Self-driving cars
- Translation
However, in the translation industry, other technical tools preceded the advent of AI. Machine translation has gained increasing acceptance over the years and is now also used in medicine and pharmacy. But what are the differences between AI-assisted translation and machine translation? To answer this question, we first need to explain what machine translation is and how it works.
What types of machine translation are there?
Three types of machine translation have evolved over the years. While early rules-based and statistical machine translation have become mostly outmoded, neural translation represents the greatest challenge to AI translation.
1. Rules-based machine translation
Rules-based machine translation, also known as the "classic" approach, is a verbatim translation approach based on information taken from source and target language dictionaries and grammars. However, this approach has proven to be very error-prone and is therefore rarely used today. Because of its limited ability to capture the subtle nuances and context of a language, rules-based machine translation has often yielded inaccurate and incomprehensible results.
2. Statistical machine translation
Statistical translation replaced rules-based translation as the dominant model in the 1990s. This approach is considered a traditional model based on the use of a large corpus of texts in both the source and target languages. Translation rules are derived from this corpus, which then serve as the basis for further translations. The special thing about statistical translation is that the machine learns the linguistic rules itself without having to be fed them manually in advance. Using statistical models and probability calculations, the machine translates based on the existing corpus. This approach led to an improvement in translation quality compared to rules-based translation and laid the foundation for further advances in machine translation technology.
3. Neural machine translation
Neural translation builds on statistical translation and has received a lot of attention in recent years. This approach requires input data that is used to train the neural network. The network learns to imitate this data, and it can be constantly improved through continuous training. A special feature of neural translation is that it teaches itself translation rules by recognizing the connections between the two languages. By using artificial neural networks, it is possible to take more complex structures and contexts into account in the translation. This approach has led to significant improvements in machine translation, and it is used by millions of people every day in the form of such services as Google Translate or DeepL, for example. Thanks to advances in technology, machine translation is becoming increasingly natural and accurate.
What is the big difference with AI translation?

Both variants have advantages and disadvantages, so the basic rule is that, depending on the intended application, it is necessary to weigh when machine translation or artificial intelligence should be used. The paragraph-based translation produced by AIs enables a better understanding of the context and a freer translation. Neural machine translation is easier to train to become an expert in a specific field, but this specialization naturally limits its use to predefined topics. In both systems, however, conceptual inconsistency is a major hurdle that can only be solved through the use of extensive and precise glossaries. Because even if the use of synonyms makes a text easy to read, the same word must always be used to mean the same thing in package inserts, manuals, or documentation. The human reviewer, the so-called post-editor, therefore remains indispensable.
Can AIs replace humans?
Due to developments in the field of artificial intelligence, more and more people are asking whether AIs can replace humans. There is no simple answer to this question. How many tasks an AI can perform depends, among other things, on the used training data and whether the AI is continuously supplied with new information. If the training data is outdated, then the AI’s knowledge is also outdated; in addition, false information found throughout the training set can be reflected in the knowledge of the model. AIs also like to use their acquired knowledge to abstract information, which means that they sometimes “invent” facts. Therefore, AIs are not infallible, which is why the generated results should always be checked carefully.
There is another challenge complicating the development and use of AIs: societal bias. People have different experiences in life, and they form different opinions on a topic and particular political outlooks. The values and prejudices of the AI developers or society at large are automatically incorporated into the programming of the AI, whether intentionally or unintentionally. The same applies to the selection and content of the training data, which is done by humans. This is a particular challenge when you consider the sheer volume of data that is needed for training.
In addition, AIs are only as good as the prompts that they receive. Depending on which prompt is entered, the result can differ greatly. Let’s illustrate our point with an example: We want the image AI Dall-E to generate an image of a house. In the first attempt, we give it the prompt "a house." In the second attempt, we offer a more detailed description of the house and the surroundings: "a photo of a red brick house with a green door and windows with green shutters and a small front yard with flowers enclosed by a white fence". Even though we offer a precise description, such as the color of the fence, for example, every image that is produced is not completely accurate.


Even if well-programmed AIs can already solve complex mathematical problems or code entire websites, there are areas where people are stronger and will probably remain stronger: Creativity, emotions, and cultural understanding can only be developed by a person and incorporated into their work.
At present, AIs provide a tool to help people in their daily work, give food for thought, or optimize processes and thus contribute to greater efficiency. AIs will probably not completely replace humans for the time being.
What are the possible uses of AIs in medicine and pharmacy?
Medicine and pharmacy are increasingly adopting digital technologies, and research is being carried out in various areas related to the use of artificial intelligence, such as, for example, nursing robots, optimization of the patient journey, and medical imaging and diagnostics. However, it is also fundamentally conceivable that artificial intelligence can be deployed for the translation of medical and pharmaceutical documents. Yet, we should proceed here with caution. Translation errors can have serious consequences in all fields of medicine. Information learned incorrectly by the AI can lead to misdiagnoses and incorrect treatments. Inaccurate translations can lead to the incorrect use of medical devices or administration of drugs. Therefore, a human being remains indispensable for ensuring the highest accuracy and quality.